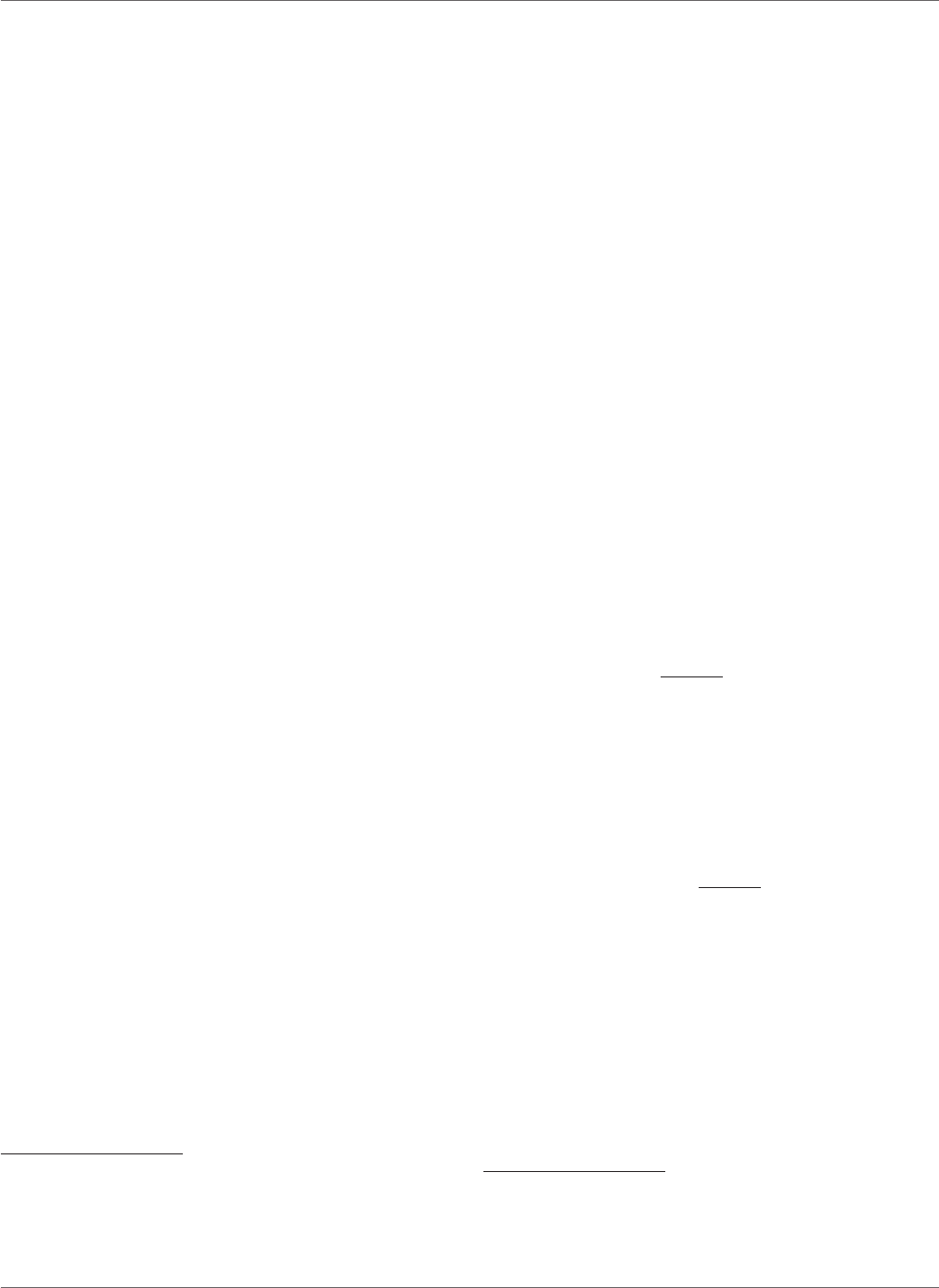
Castaneda and Saygili State-Wide Shelter-in-Place Order and Social Distancing in Texas
that encourage and sometimes force social distancing
2
. In the
United States, the state-wide shelter-in-place policies are the most
common of such policies. Shelter-in-place orders (SIPO) require
residents to remain home for all but essential activities such
as purchasing food or medicine, caring for others, exercise, or
traveling for employment deemed essential. Between March 19
and April 20, 2020, 40 states and the District of Columbia adopted
SIPOs (
3).
A fast-growing body of literature explores the potential
impacts of stay-at-home orders on various indicators from
the COVID-19 hospitalizations, cases, and fatalities to their
implications for mental health (4, 5), physical he a lth (6, 7), and
domestic violence (8–10). Some studies find that stay-at-home
orders are associated with a slower growth rate of hospitalization
(11, 12). Closer to our study are the ones t hat focus on COVID
cases and deaths. (13) analyzes the US data from March 1 , 2020,
to April 27, 2020, and find that adoption of government-imposed
social distancing measures reduced the da ily growth rate by 5.4
percentage points after 1–5 days, 6.8 after 6–10 days, 8.2 after
11–15 days, and 9.1 after 16–20 days. Similarly, (
3) work with
data from all the states from March 8, 2020, to April 17, 2020.
Using daily state-level social distancing data from SafeGraph and
a difference-in-differences approach, they find that adoption of a
shelter-in-place order (SIPO) is associated with a 5–10% increase
in the rate at which state residents remained in their homes full-
time. Also, using daily state-lev el coronavirus case data collected
by the CDC, th ey find that approximately 3 weeks following the
adoption of a SIPO, cumulative COVID-19 cases fell by 44%.
Similar to our paper, (14) focus on a particular state, namely
California, to estimate the impact of the state-wide SIPO on the
COVID-19 cases and deaths.
We focus on a large state, Texas. Our data span almost
4 months, which allows us to see better the evolution of the
pandemic during as well as after the expiry of the SIPO. The
fact that Texas is one of the relatively early states to start t h e
reopening and experiences a post-opening surge in t he number
of cases makes it an interesting case to study closely. We use daily
data from March 1, 2020 to June 27, 2020, to study t h e evolution
of the COVID-19 pandemic in 254 Texas counties. We analyze
the impact of the statewide shelter-in-place order issued on April
2, 2020, on the measures of social distancing, and consequently,
on t h e COVID-19 cases and dea ths.
2. MATERIALS AND METHODS
2.1. Data
The data for this study comes from the Unfolded Studio website
(https://covid19.unfolded.ai/). The website compiles data from
various sources. The COVI D-19 cases and fatalities data are
from the New York Times
3
. Social distancing measures are
2
Social distancing, also called “physical distancing” is defined by the CDC as
keeping a safe space (at le ast 6 feet) between yourself and other people who are
not from your household.
3
The data are consistent with the count of cases and fatalities from the CDC and
John Hopkins University.
from SafeGraph
4
. County-level population data are from the US
Census. SafeGraph’s social distancing data are generated using a
panel of GPS pings from anonymous mobile devices. The data
determine the common nighttime locat ion of each device and
call that location “home” (approximately a 153 by 153 m area).
The shelter-in-place percentage reported in the Unfolded data
shows the percentage of the population who stayed at home on a
day. Finally, we use the National Association of Counties’ (NACo)
county explorer to get county-level COVID 19 related policy
responses. Our dataset is daily and at the county level.
2.2. Methods
2.2.1. Theoretical Background
We provide a basic description of the theoretical foundations for
the spread of the disease. Although the model of a pandemic
is not strictly exponential, the exponential growth model may
provide a good approximation at the beginning of the pandemic
and is implicitly used in much of the literature.
The discrete-time version of the exponential growth model is
described by the equation
5
:
c
t
= c
0
(1 + δ)
t
(1)
where c
t
denotes the total number of cases at time t, δ represents
the growth rate, and c
0
is the number of cases at time z ero.
The parameter of interest is δ, the rate of growth of the process,
which can be interpreted as the number of infected individuals
by one infected individual in a day. Rearranging Equation (1), we
can write
c
t+1
− c
t
c
t
= δ . (2)
Given a sample
{
c
t
}
of observations, we are interested in
estimating the growth rate and the effect of a policy variable (T
t
)
on t he growth rate. This can be done by estimating the equation
y
t
= β
1
+ α
1
T
t
+ u
t
(3)
where
y
t
=
c
t+1
− c
t
c
t
(4)
where y
t
is the growth rate, β
1
is the estimated growth rate before
the policy, and β
1
+α
1
represents the growth rate after the policy,
with α
1
being t he effect of the policy.
The model we present provides a good approximation for
the evolution of the number of cases. Fatalities, however, are
much harder to model. Similar to cases, we analyze the growth
rate of deaths to provide a complete picture. However, we are
aware of the problems associated with fitting the same type of
model for cases and fatalities. First, social distancing may have
different effects on the growth of cases and deaths. In particular,
social distancing measures are more likely to show a bigger and
4
Several studies rely on SafeGraph for social distancing measures. See (
3, 14–17)
among many others.
5
The continuous-time version yields similar conclusions. We stick to the discrete
version for expositional simplicity.
Frontiers in Public Health | www.frontiersin.org 2 November 2020 | Volume 8 | Article 596607