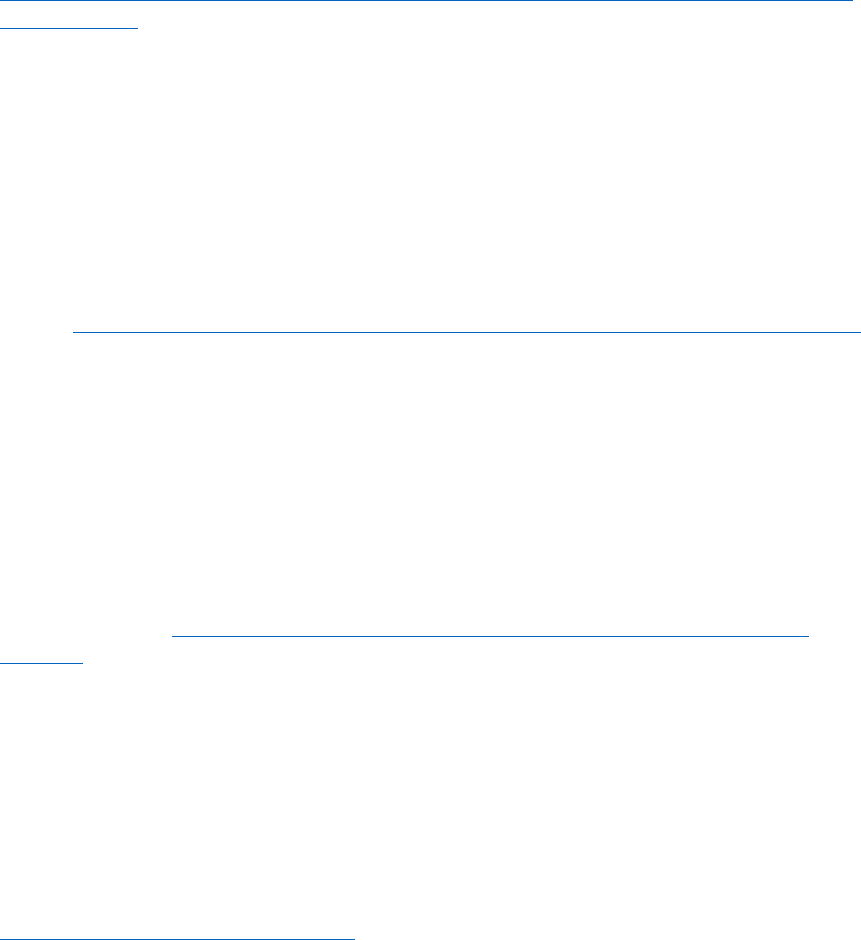
21
References
Allday, Erin. 2020. “Bay Area Orders ‘Shelter in Place,’ Only Essential Businesses Open in 6
Counties.” San Francisco Chronicle, March 16. Available at:
https://www.sfchronicle.com/local-politics/article/Bay-Area-must-shelter-in-place-Only-
15135014.php
Baek, ChaeWon, Peter B. McCrory, Todd Messer, and Preston Mui. 2020. “Unemployment
Effects of Stay-at-Home Orders: Evidence from High Frequency Claims Data.” Institute
for Research on Labor and Employment Working Paper No. 101-20.
Bai, Yan, Lingsheng Yao, Tao Wei, Fei Tian, Dong-Yan Jin, Lijuan Chen, and Meiyun Wang.
2020. "Presumed asymptomatic carrier transmission of COVID-19." JAMA, 323(14):
1406-1407.
Bertrand, Marianne, Esther Duflo, and Sendhil Mullainathan. 2004. “How much should we trust
differences-in-differences estimates?” The Quarterly Journal of Economics, 119(1): 249-
275.
Centers for Disease Control. 2020. “COVID-19 How to Protect Yourself and Others.” Available
online: https://www.cdc.gov/coronavirus/2019-ncov/prevent-getting-sick/prevention.html
Courtemanche, Charles, Joseph Garuccio, Anh Le, Joshua C. Pinkston, and Aaron Yelowitz.
2020a. “Strong Social Distancing Measures In The United States Reduced The COVID-
19 Growth Rate.” Health Affairs, in press.
Courtemanche, Charles J., Joseph Garuccio, Anh Le, Joshua C. Pinkston, and Aaron Yelowitz.
2020b. "Did Social-Distancing Measures in Kentucky Help to Flatten the COVID-19
Curve?" Institute for the Study of Free Enterprise Working Paper 2020-4.
Dave, Dhaval M., Andrew I. Friedson, Kyutaro Matsuzawa, and Joseph J. Sabia. 2020. “When
Do Shelter-In-Place Orders Fight COVID-19 Best? Policy Heterogeneity across States
and Adoption Time.” National Bureau of Economic Research Working Paper No. 27091.
Defense Casualty Analysis System. 2008. “Vietnam War U.S. Military Fatal Casualty Statistics.”
Available online: https://www.archives.gov/research/military/vietnam-war/casualty-
statistics
Friedson, Andrew I., Drew McNichols, Joseph J. Sabia, and Dhaval Dave. 2020. “Did
California’s Shelter-in-Place Order Work? Early Coronavirus-Related Public Health
Effects.” National Bureau of Economic Research Working Paper No. 26992.
Goodman-Bacon, Andrew. 2018. “Difference-in-Differences with Variation in Treatment
Timing.” National Bureau of Economic Research Working Paper No. 25108.
Holshue, Michelle L., Chas DeBolt, Scott Lindquist, Kathy H. Lofy, John Wiesman, Hollianne
Bruce, Christopher Spitters, et al. 2020. “First case of 2019 novel coronavirus in the
United States.” New England Journal of Medicine, 382: 929-936.
Johns Hopkins University. 2020. “COVID-19 Case Tracker.” Available online:
https://coronavirus.jhu.edu/map.html
Lauer, Stephen A., Kyra H. Grantz, Qifang Bi, Forrest K. Jones, Qulu Zheng, Hannah R.
Meredith, Andrew S. Azman, Nicholas G. Reich, and Justin Lessler. 2020. “The
Incubation Period of Coronavirus Disease 2019 (COVID-19) from Publicly Reported
Confirmed Cases: Estimation and Application.” Annals of Internal Medicine