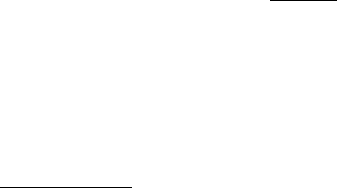
The Annals of Applied Probability
2020, Vol. 30, No. 5, 2491–2515
https://doi.org/10.1214/20-AAP1564
© Institute of Mathematical Statistics, 2020
ASYMPTOTIC THEORY OF SPARSE BRADLEY–TERRY MODEL
B
Y RUIJIAN HAN
*
,ROUGANG YE
†
,CHUNXI TAN
‡
AND KANI CHEN
§
Department of Mathematics, Hong Kong University of Science and Technology,
*
†
‡
§
The Bradley–Terry model is a fundamental model in the analysis of net-
work data involving paired comparison. Assuming every pair of subjects in
the network have an equal number of comparisons, Simons and Yao (Ann.
Statist. 27 (1999) 1041–1060) established an asymptotic theory for statistical
estimation in the Bradley–Terry model. In practice, when the size of the net-
work becomes large, the paired comparisons are generally sparse. The spar-
sity can be characterized by the probability p
n
that a pair of subjects have at
least one comparison, which tends to zero as the size of the network n goes to
infinity. In this paper, the asymptotic properties of the maximum likelihood
estimate of the Bradley–Terry model are shown under minimal conditions of
the sparsity. Specifically, the uniform consistency is proved when p
n
is as
small as the order of (log n)
3
/n, which is near the theoretical lower bound
log n/n by the theory of the Erd
˝
os–Rényi graph. Asymptotic normality and
inference are also provided. Evidence in support of the theory is presented in
simulation results, along with an application to the analysis of the ATP data.
1. Introduction. Is Roger Federer a better tennis player than John McEnroe? Is research
article A more influential than research article B, among a collection of all research articles in
a scientific field? Is webpage A more important than webpage B, among the existing millions
of webpages? Is person A more popular than person B in a large social network, such as
Twitter users? These questions may be answered by analysis of paired comparison data in a
network. The paired comparison may be in terms of head-to-head match outcomes, citation
of a research article by another, a webpage containing a link of another webpage, a user
retweeting the tweet of another user, etcetera. When the size of the network, such as a total
number of webpages, becomes large, paired comparisons are generally sparse. The sparsity
may be described in different ways, such as the total number of observed comparisons divided
by the total number of subjects in the network. Throughout this paper, the size of the network
in study is denoted as n, and we assume any pair has a comparison with probability p
n
.The
degree of sparsity is then characterized by the size of p
n
, the smaller the sparser.
For paired comparison, the Bradley–Terry model (Bradley and Terry (1952)) is one of the
most commonly used models. Consider n subjects in a network. Subject i has merit u
i
for
i =0,...,n−1, where u
i
∈R and u
i
> 0. The Bradley–Terry model assumes the probability
that subject i defeats j as
(1.1) p
ij
=
u
i
u
i
+u
j
,i,j=0,...,n− 1;i = j.
The generalizations of the Bradley–Terry model can be seen in, for example, Luce (1959),
Rao and Kupper (1967)andAgresti (1990), among many others. For estimation of the merits
based on a set of paired comparison data, the maximum likelihood estimation (MLE) is a
common choice. It is much desired to justify the asymptotic properties of the MLE, particu-
larly when the comparisons are sparse. A distinct feature of this problem is that the number
Received June 2019; revised October 2019.
MSC2020 subject classifications. Primary 60F05; secondary 62E20, 62F12, 62J15.
Key words and phrases. Bradley–Terry model, sparsity, uniform consistency, asymptotic normality, maximum
likelihood estimation.
2491