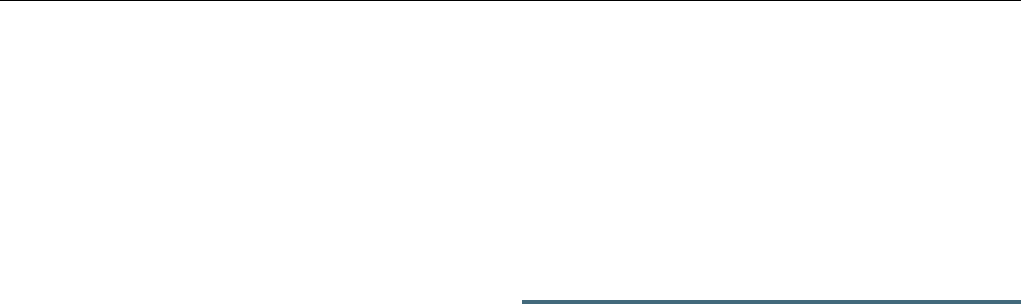
use accelerometer data to assist HR estimation [18]. There is
some evidence that gyroscopes could be used [19] to reduce
PPG signal noise, so we are likely to see more devices in the
future equipped with PPG sensors. To further enrich the PA
data collection, some devices have a built in global positioning
system (GPS) receiver. This is especially true for high-end
fitness trackers and sports watches specifically targeting
physically active people. With a GPS, it is possible to track
more data, including position, speed, and altitude.
Algorithms and Mobile Apps
Raw data from sensors must be converted into readable metrics
to be meaningful for the user. Many devices only display a
limited set of metrics directly on the device (eg, today’s step
count or current HR) and rely on an accompanying mobile app
to show the full range of available metrics (eg, historic daily
step count and detailed HR data). Although the physical sensors
in these devices are very similar, the algorithms that interpret
sensor output are unique for most vendors. These algorithms
are often company secrets, and they can be changed without
notice. In addition, the quality and supported features of the
accompanying mobile apps varies, and the total user experience
will therefore differ. Each additional sensor included in a device
can be used to add additional types of metrics for the user or
supply internal algorithms with additional data to improve
accuracy of already available metric types. However, additional
sensors affect price and power consumption.
Device Types
There are many similarities between different types of devices,
and they may be difficult to categorize. We will use the term
wearable in this paper as a common term for wrist-worn devices
that can track and share PA data with a mobile phone.
A smartwatch is a wrist-worn device that, mostly, acts as an
extension to a mobile phone and can show notifications and
track PA and related metrics. Modern smartwatches often
include a touch screen and can support advanced features and
display high resolution activity trends [15]. Fitness trackers (ie,
smart band or fitness band), normally worn on the wrist or hip,
are devices more dedicated to PA tracking. A fitness tracker is
typically cheaper than a smartwatch because of less expensive
hardware and often fewer sensors. Due to this, it generally also
has better battery life and a limited interface for displaying
tracking results [15].
Other terms are also used, for example, sports watch and GPS
watch, which can be considered merges between smartwatches
and fitness trackers. In addition, there are hybrid watches (ie,
hybrid smartwatches) that have a traditional clockwork and
analogue display that have been fitted with an accelerometer.
An accompanying mobile app is needed to access most data,
but daily step counts are often represented as an analogue gauge
on the watch face.
Wearable Usage Scenario
Wearables come forward as a new alternative to tracking PA
in research (compared with, eg, ActiGraph), especially when it
is desired to collect measurements for a prolonged period of
time. In an intervention study, continuous data collecting from
wearables would allow researchers to better track changes in
PA and adjust the intervention accordingly. Wearables can also
be used in epidemiological research as a tool for tracking PA
for an extended period. This could reveal detailed PA changes
in a population over time. In both scenarios, there are several
potential important requirements to consider when choosing a
device for the study, including usability, battery life, price,
accuracy, durability, look and feel, and data access possibilities.
Methods
Search Strategies
Brands, Devices, and Sensors
We searched six databases to create a list of relevant wearable
devices: The Queen’s University’s Wearable Device Inventory
[20], The Vandrico Wearables database [21], GsmArena [22],
Wearables.com [23], SpecBucket [24], and PrisGuide [25,26].
We only used publicly available information when comparing
devices. We did the search from May 15, 2017 to July 1, 2017.
We identified wearables in two steps. In step one, we identified
and searched the six defined databases. In step two, we extracted
all brands from the list of devices identified in step one and
examined brand websites for additional devices. If we found
the same device in several databases with conflicting
information, we manually identified the correct information
from the device’s official website or other online sources (eg,
Wikipedia and Google search). We removed duplicates and
devices not fitting the inclusion criteria.
Brand Usage in Research
We searched Ovid MEDLINE on September 30, 2017 to
determine how often the most relevant brands were used in
previous studies. For each search, we performed a keyword
search with no limitations set. We divided our findings into
validation and reliability studies and data collection studies.
To decide which brand to consider most relevant, we did two
sets of searches. In the first set, we created a brand-specific
keyword search for brands that were (1) One of the five most
sold brands in 2015 or 2016 or (2) Had released 10 or more
unique devices. From the resulting list of articles, we screened
title, abstract, and the method section. This screening was done
to (1) Exclude articles out of scope and (2) To identify additional
brands used in these studies. We compiled a list of these brands
and performed a second set of searches, one for each new
identified brand. Eleven brands were finally included. The
specific keyword search used for each brand is given in the
Results section where we summarize our findings.
We also searched the US National Library of Medicine database
of clinical studies through the ClinicalTrials website, using the
same 11 keyword searches, to determine brand usage in ongoing
projects. One author did the articles screening, as well as the
projects description screening in ClinicalTrials.
Brand Developer Possibilities
To determine how relevant a specific brand is when planning
a new research project, we reviewed the 11 identified brands
and considered available developer options, supported mobile
J Med Internet Res 2018 | vol. 20 | iss. 3 | e110 | p. 3http://www.jmir.org/2018/3/e110/
(page number not for citation purposes)
Henriksen et alJOURNAL OF MEDICAL INTERNET RESEARCH
XSL
•
FO
RenderX