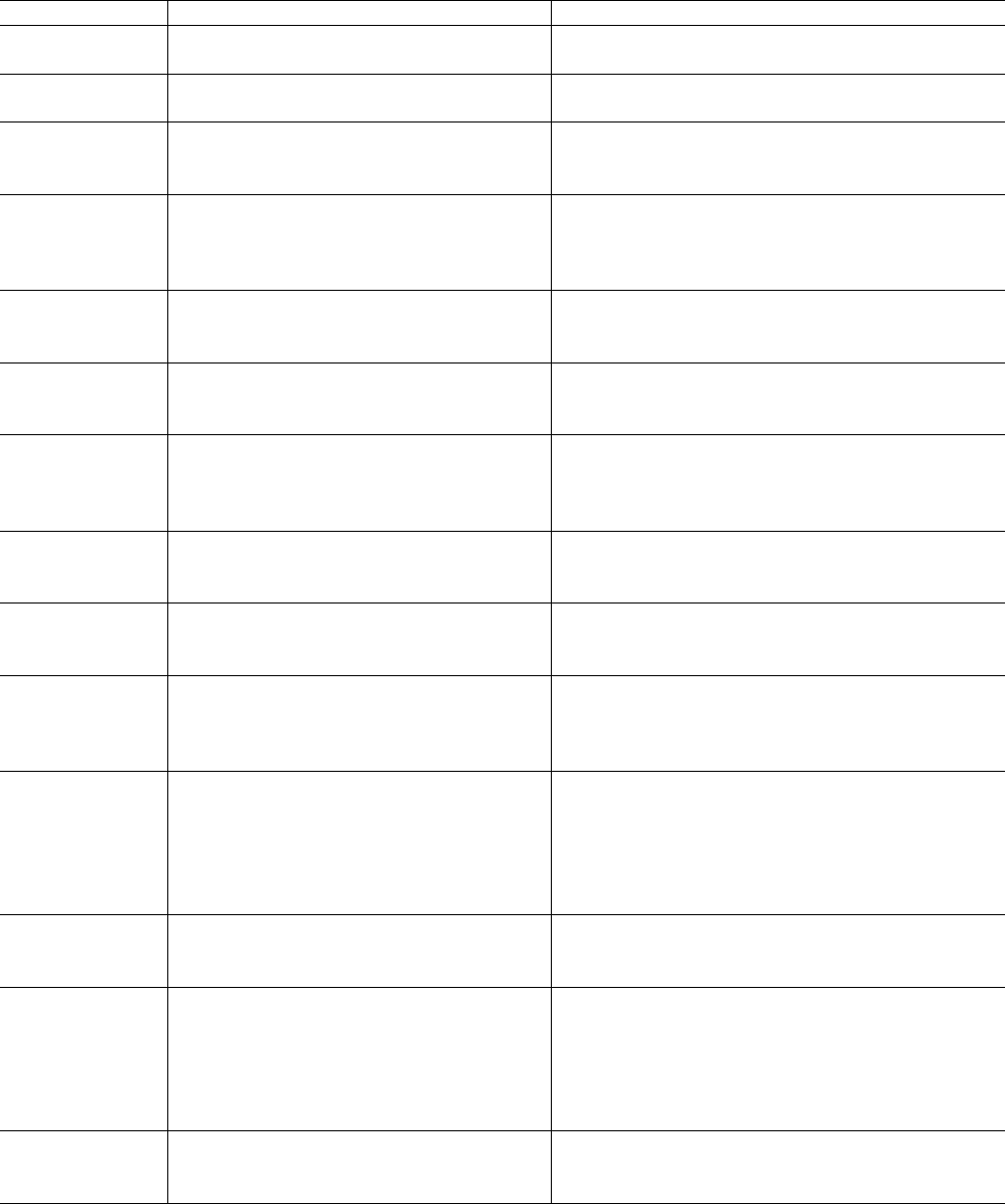
Table 7: Description of Error Rule Categories with Examples
Category Description Examples
American En-
glish Phrases
Sentence favors the American English
spelling of words.
apologize, catalog, civilization, defense
British English,
Oxford Spelling
Sentence favors the British English
spelling of words.
apologise, catalogue, civilisation, defence
Capitalization Rules about detecting uppercase words
where lowercase is required and vice
versa.
This house is old. it was built in 1950.
I really like Harry potter.
Collocations A collocation is made up of two or more
words that are commonly used together in
English. This refers to an error in this
type of phrase.
Undoubtedly, this is the result of an extremely dy-
namic development of Lublin in the recent years.
I will take it in to account.
It’s batter to be save then sorry.
Commonly Con-
fused Words
Words that are easily confused, like ’there’
and ’their’ in English.
I have my won bed.
Their elicit behavior got the students kicked out of
school.It’s the worse possible outcome.
Grammar Violations related to system of rules that
allow us to structure sentences.
Tom make his life worse.
A study like this one rely on historical and present
data.This is best way of dealing with errors.
Miscellaneous Miscellaneous rules that don’t fit else-
where.
This is best way of dealing with errors.
The train arrived a hour ago.
It’s nice, but it doesn’t work. (inconsistent apostro-
phes)
Nonstandard
Phrases
I never have been to London.
List the names in an alphabetical order.
Why would a man all of the sudden send flowers?
Possible Typo Spelling issues. It’a extremely helpful when it comes to homework.
We haven’t earned anything.This is not a HIPPA
violation.
Punctuation Error in the marks, such as period,
comma, and parentheses, used in writing
to separate sentences and their elements
and to clarify meaning.
"I’m over here, she said.
Huh I thought it was done already.
The U.S.A is one of the largest countries.
Redundant
Phrases
Redundant phrases contain words that
say the same thing twice. When one of the
words is removed, the sentence still makes
sense. Sometimes the sentence has to be
slightly restructured, but the message re-
mains the same.
We have more than 100+ customers.
He did it in a terrible way.
The money is sufficient enough to buy the sweater.
Semantics Logic, content, and consistency problems. It allows us to both grow, focus, and flourish.
On October 7, 2025 , we visited the client.This was
my 11nd try.
Style General style issues not covered by other
categories, like overly verbose wording.
Moreover, the street is almost entirely residential.
Moreover, it was named after a poet.
Doing it this way is more easy than the previous
method.
I’m not very experienced too.
Anyways, I don’t like it.
Typography Problems like incorrectly used dash or
quote characters.
This is a sentence with two consecutive spaces.
I have 3dogs.The price rose by $12,50.
I’ll buy a new T—shirt.
35