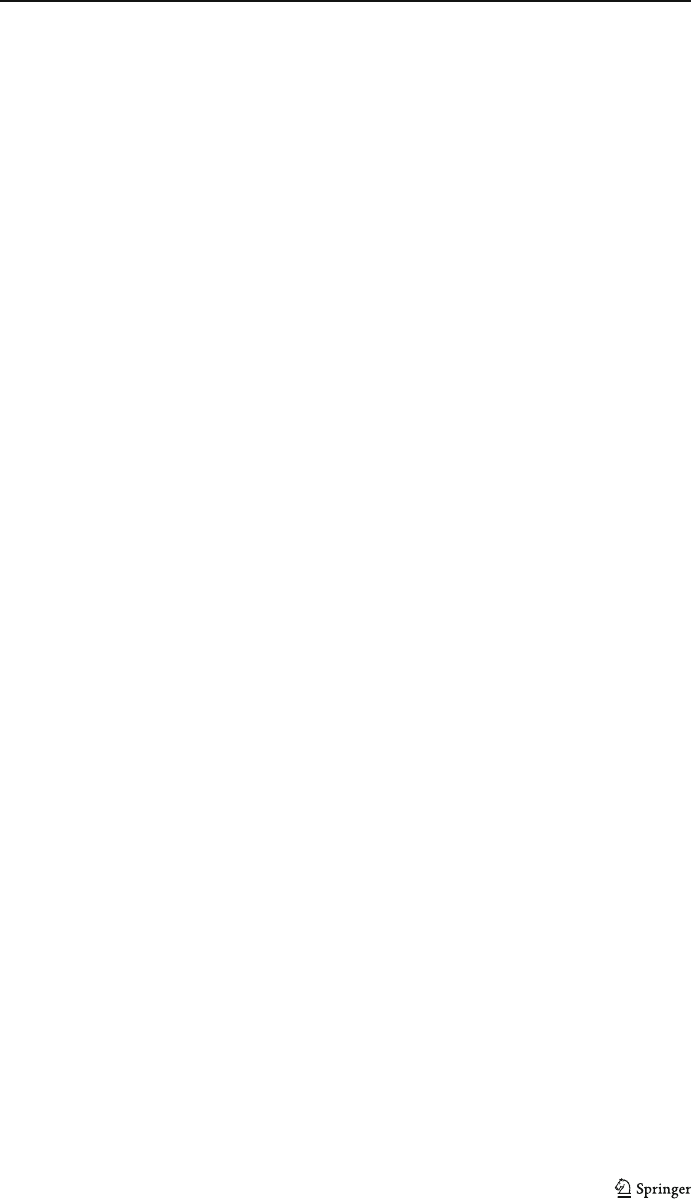
Barlow, D. H., Nock, M. K., & Hersen, M. (2009). Single case experimental designs: strategies for studying
behavior change (3rd ed.). Upper Saddle River: Pearson.
Bowen, R. (1992). Graph it! How to make, read, and interpret graphs. Englewood Cliffs: Prentice-Hall.
Carr, J. E., & Britton, L. N. (2003). Citation trends of applied journals in behavioral psychol-
ogy: 1981–2000 . Journal of Ap plied Behavior Analysis, 36,113–117. doi:10.1901/j aba.2003.
36-113.
Catania, A. C. (1998). Learning (4th ed.). Englewood Cliffs: Prentice-Hall.
Cleveland, W. S. (1984a). Graphs in scientific publications. The American Statistician, 38,261–269. doi:10.
1080/00031305.1984.10483223.
Cleveland, W. S. (1984b). Graphical methods for data presentation: full scale breaks, dot charts, and multibased
logging. The American Statistician, 38, 270–280. doi:10.1080/00031305.1984.10483224.
Cleveland, W. S. (1993). Visualizing data. Summit: Hobart Press.
Cleveland, W. S. (1994). The elements of graphing data. Summit: Hobart Press.
Cleveland, W. S., & McGill, R. (1985). Graphical perception and graphical methods for analyzing scientific data.
Science, 229(4716), 828–833. doi:10.1126/science.229.4716.828.
Cooper, J. O., Heron, T. E., & Heward, W. L. (2007). Applied behavior analysis (2nd ed.). Upper Saddle River:
Pearson Prentice Hall.
Critchfield, T. S. (2002). Evaluating the function of applied behavior analysis: a bibliometric analysis. Journal of
Applied Behavior Analysis, 35, 423–426. doi:10.1901/jaba.2002.35-423.
Department of the A rmy. (2010). Standards of statistical presentation. Washington DC: Department of the
Army.
Ferster, C. B., & Skinner, B. F. (1957). Schedules of reinforcement. New York: Appleton.
Few, S. (2009). Now you see it: simple visualization techniques for quantitative analysis. Oakland: Analytics
Press.
Gast, D. L. (2010). Single subject research methodology in behavioral sciences. New York: Routledge.
Gast, D. L., & Spriggs, A. D. (2010). Visual analysis of graphic data. In D. L. Gast (Ed.), Single subject research
methodology in behavioral sciences (pp. 166–233). New York: Routledge.
Giesecke, F. E., Hill, I. L., Spencer, H. C., Mitchell, A. E., Dygdon, J. T., Novak, J. E., Lockhart, S. E., &
Goodman, M. (2012). Technical drawing with engineering graphics (14th ed.). Upper Saddle River:
Pearson.
Gutentag, S., & Hammer, D. (2000). Shaping oral feeding in a gastronomy tube-dependent child in natural
settings. Behavior Modification, 24,395–410. doi:10.1 177/0145445500243006.
Harris,R.L.(1999).Information graphics: a comprehensive illustrated reference. New York: Oxford University
Press.
International Organization for Standardization (2014, June 4). What are standards? Retrieved from htt
p://www.
iso.org/iso/about/discover-iso_meet-iso/about.htm
Johnston, J. M., & Pennypacker, H. S. (1980). Strategies and tactics of human behavioral research. Hillsdale:
Erlbaum.
Johnston, J. M., & Pennypacker, H. S. (2009). Strategies and tactics of behavioral resear ch (3rd ed.). New York:
Routledge.
Kangas, B. D., & Cassidy, R. N. (2010). Requiem for my lovely. Journal of the Experimental Analysis of
Behavior, 95, 269. doi:10.1901/jeab.2011.95-269.
Katzenberg, A. C. (1975). How to draw graphs. Chicago: R.R. Donnelley & Sons Company.
Kazdin, A. E. (2011). Single-case research designs: methods for clinical and applied settings (2nd ed.). New
York: Oxford University Press.
Kennedy, C. H. (2005). Single-case designs for educational research. Boston: Allyn & Bacon.
Kriglstein, S., Pohl, M., & Smuc, M. (2014). Pep up your time machine: recommendations for the design of
information visualizations of time-dependent data. In W. Huang (Ed.), Handbook of human centric
visualization (pp. 203–225). New York: Springer.
Kubina, R. M., Kostewicz, D. E., & Datchuk, S. M. D2008]. An initial survey of fractional graph and
table area in behavioral journals. Behavior Analyst, 31,61–66. http://www.ncbi.nlm.nih.gov/p mc/
articles/PMC2395347/.
Lane, J. D., & Gast, D. L. (2014). Visual analysis in single case experimental design studies: brief review and
guidelines. Neur opsychological Rehabilitation: An International Journal, 24(3–4), 445–463. doi:10.1080/
09602011.2013.815636.
Lattal, K. A. (2004). Steps and pips in the history of the cumulative recorder. Journal of Experimental Analysis of
Behavior, 82, 329–355. doi:10.1901/jeab.2004.82-329.
Malott, R. W., & Shane, J. T. (2014). Principles of behavior (7th ed.). Upper Saddle River: Pearson.
Mayer, G. R., Sulzer-Azaroff, B., & Wallace, M. (2014). Behavior analysis for lasting change (3rd ed.).
Cornwall-on-Hudson: Sloan.
Educ Psychol Rev (2017) 29:583–598 597